Welcome to Tianyi’s Homepage
I received my bachelor’s degree(Honors) in Artificial Intelligence from College of Computer Science and Technology, Zhejiang University in June 2024. I’m also a member of Turing Class at Chu Kochen Honors College. I’m currently in my gap year to further pursue my research interests and search for potential opportunities.
Previously, I was an intern working on Open-Sora in HPC-AI Tech. Before that, I spent a wonderful summer as a research intern advised by Prof. Yang You and Dr. Kai Wang at NUS HPC-AI Lab, focusing on diffusion models and video generation. Earlier in my academic journey, I was fortunate to work with Prof. Junbo Zhao and Prof. Yang Yang at Zhejiang University, focusing on the evaluation of LLMs and active learning on graphs, respectively.
My research interest lies in but is not limited to multimodality machine learning, data science and AI foundations. My goal is to conduct foundational scientific research to improve the performance, efficiency, generalizability and versatility of machine learning models, as well as deepen our comprehension of the underlying principles and scientific facts of neural networks.
Recently I’m working on diffusion models, multimodality representation learning/matching, and sparse models.
Feel free to contact me via email: aicfw.li@gmail.com . I’ll become very happy to chat and collaborate.
Research
- Implicit Semi-auto-regressive Image-to-Video Diffusion
- Submitted to ICLR’2024
- Tianyi Li, Kai Wang, Ziheng Qin, David Junhao Zhang, Tianle Zhang, Junbo Zhao, Mike Zheng Shou, Yang You
- We present a novel temporal recurrent look-back approach for modeling video dynamics, leveraging prior information from the first frame (provided as a given image) as an implicit semi-auto-regressive process. Conditioned solely on preceding frames, our approach achieves enhanced consistency with the initial frame, thus avoiding unexpected generation results.
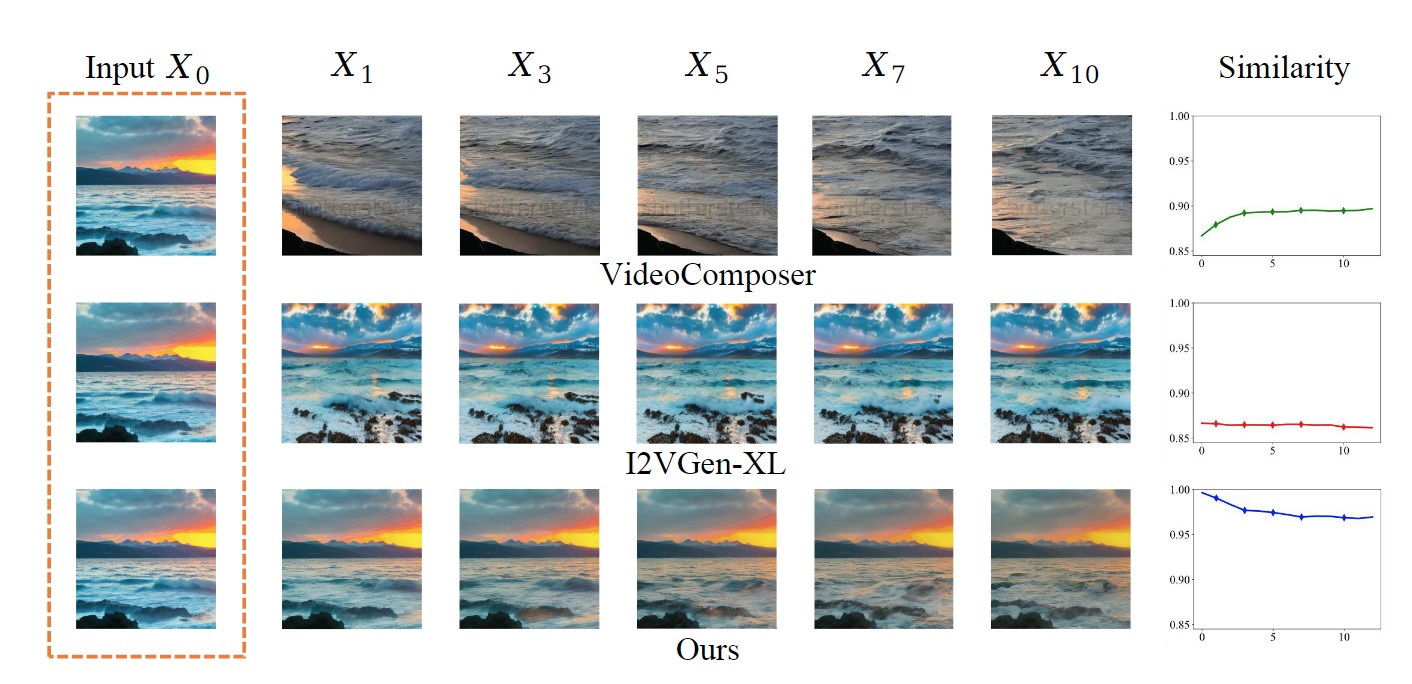
- Assessing Hidden Risks of LLMs: An Empirical Study on Robustness, Consistency, and Credibility
- ArXiv Preprint
- Wentao Ye, Mingfeng Ou, Tianyi Li, Yipeng chen, Xuetao Ma, Yifan Yanggong, Sai Wu, Jie Fu, Gang Chen, Haobo Wang, Junbo Zhao
- We propose an automated workflow and conduct over a million queries to the main-stream LLMs including ChatGPT, LLaMA, and OPT. We also introduce a novel index associated with a dataset that roughly decides the feasibility of using such data for LLM-involved evaluation. We draw several conclusions that are quite uncommon in current LLM community.
- Paper / Code
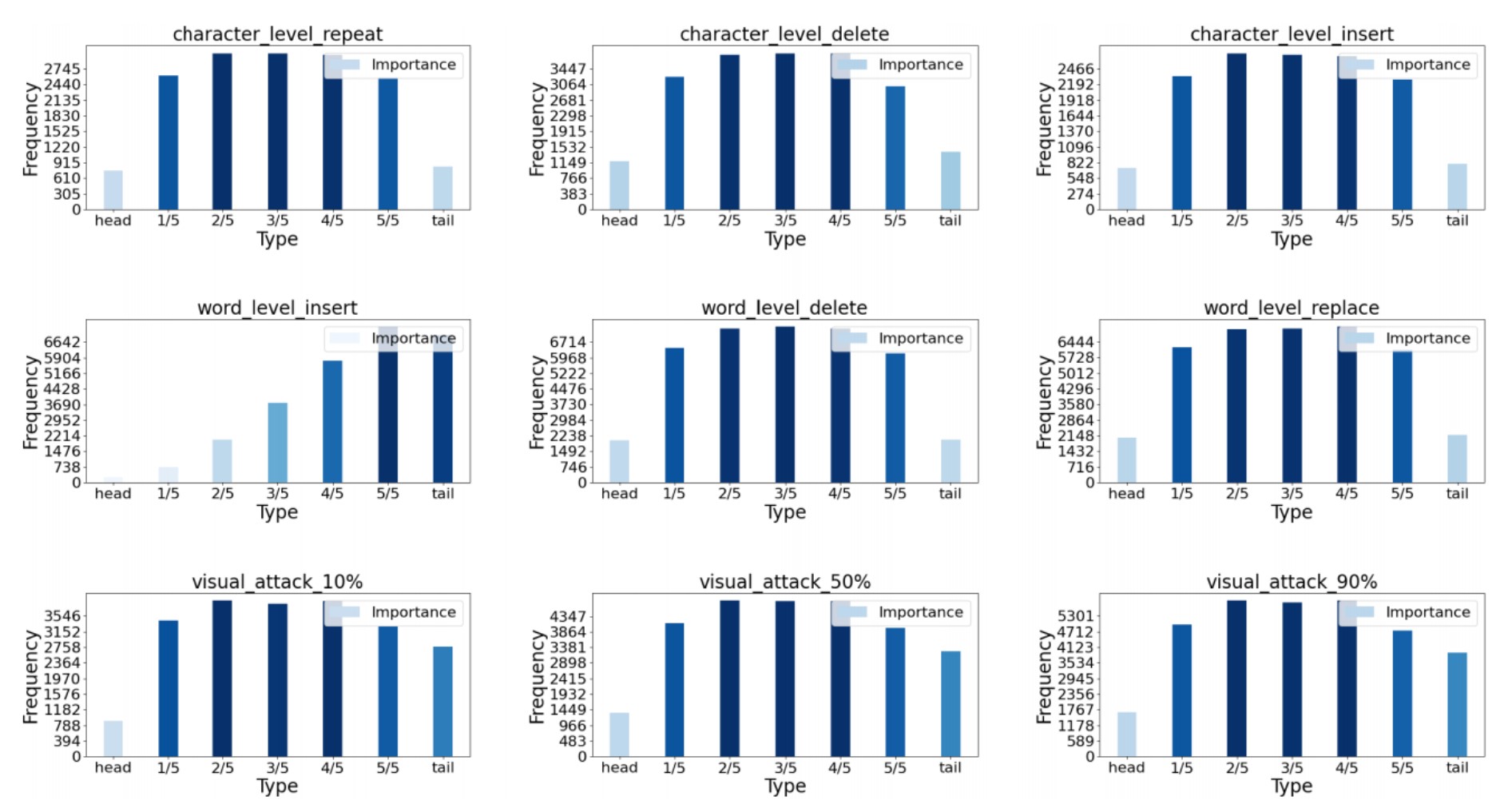
- Structure is All You Need? Label Efficiency MAX with Simple Feature-free Graph Active Learning Paradigm
- Rejected by NeurIPS’2023, refining
- Tianyi Li, Renhong Huang, Jiayu Liu, Tianxin Zheng, Yang Yang, Jiarong Xu
- We propose a novel graph active learning paradigm that is feature-free and only requires the graph structure.
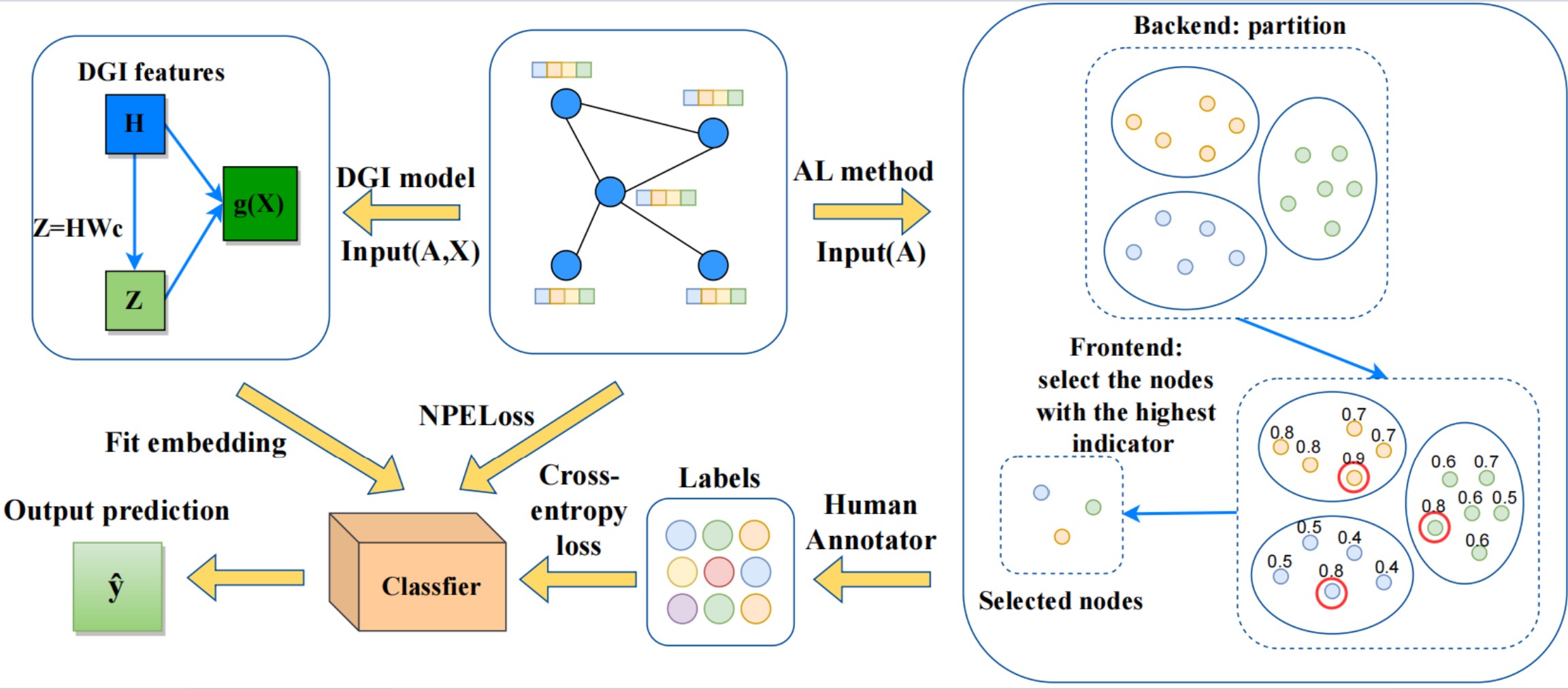
Honors and Awards
- Outstanding Graduate of Zhejiang University
- Outstanding Undergraduate Thesis of Zhejiang University
- 2nd Scholarship of Zhejiang University